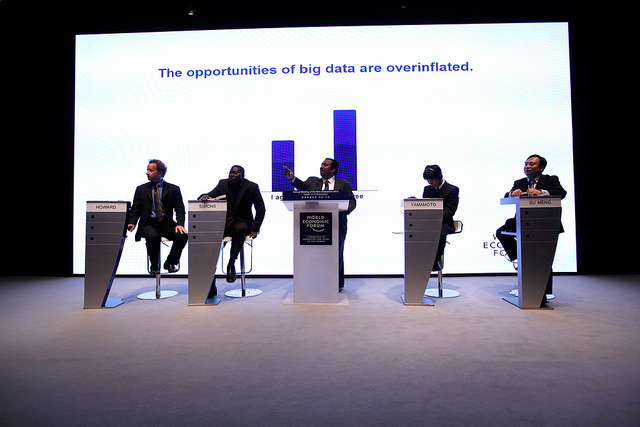
Over the past few decades, researchers in a variety of fields have had to come to grips with analyzing massive data sets. These can be generated intentionally, through things like astronomy surveys and genome sequencing, or they can be generated incidentally—through things like cell phone records or game logs.
The developments of algorithms that successfully pull information from these masses of data has led some of the more enthusiastic proponents of big data to argue that it will completely change the way science is done (one even argued that big data made the scientific method obsolete). In today's issue of Science, however, a group of scientists throw a bit of cold water on the big-data hype, in part by noting that one of the publicly prominent examples of massive data analysis, Google Flu Trends, isn't actually very good.
Not so trendy
Their analysis builds on an earlier report from Nature News that highlights a few clear failures of Google Flu Trends. The service is meant to give real-time information on seasonal flu outbreaks by tracking a series of search terms that tend to be used by people who are currently suffering from the flu. This should provide a bit of lead time over the methods used in the US and abroad, which aggregate monitoring data from a large number of healthcare facilities. Those are considered the definitive measurements, but the testing and data aggregation take time, while Flu Trends can be updated in near real time.
The problem is that Flu Trends has gotten it badly wrong in at least two cases. The reason for these errors is remarkably simple: the flu was in the news, and people were therefore more interested and/or concerned about its symptoms. Use of the key search terms rose, and, at some points, Google Flu Trends predicted double the number of infected people than were later revealed to exist by the Centers for Disease Control data. (One of these cases was the global pandemic of 2009; the second an early and virulent start to the season in 2013.)
On its own, this isn't especially damning. But the authors note that flu trends have consistently overestimated actual cases, estimating high in 93 percent of the weeks in one two-year period. You can do just as well by taking the lagging CDC data and putting it into a model that contains information about past flu dynamics. And, unlike the Flu Trends algorithm, they point out that this sort of model can be improved.
In describing their system, the Flu Trends engineers have said that they started by identifying a series of search terms that correlated with CDC data. They then had to exclude a bunch of search terms that correlate with flu searches simply because they follow the same seasonality (high school basketball was apparently one of them). And the remaining terms? They've never actually been described in full, even as Google engineers have added revisions to the system. That means that Flu Trends results are fundamentally irreproducible, and nobody outside of Google could ever improve the system.
Complicating matters further, Google changes its search behavior and results in various ways for reasons that have nothing to do with flu trends, and those feed back into user behavior in complicated ways. The company has also engaged in constant warfare with people who want to game its system, a problem it shares with other commercial sources of big data. All of these factors can make the real goal of big data analyses—getting at some underlying feature of reality—a tricky prospect.
Thinking big
The researchers note that none of this means that Google Flu Trends is useless. It would be more useful if it were reproducible, but even without that, it serves as a helpful addition to the CDC numbers. And, although the piece reads like a bit of a takedown of Flu Trends, the authors' target is something larger, something they call "big data hubris."
This they define as "the often implicit assumption that big data are a substitute for, rather than a supplement to, traditional data collection and analysis."
The problem they identify resulting from this form of hubris is that it's relatively easy to use big data to identify eye-catching and publicity-generating correlations. It's much harder to turn these correlations into something that's scientifically actionable, and harder still to do the actual experiments that reach a scientifically valid conclusion. (As the authors put this, "The core challenge is that most big data that have received popular attention are not the output of instruments designed to produce valid and reliable data amenable for scientific analysis.")
Put another way, it's not uncommon to hear the argument that "computer algorithms have reached the point where we can now do X." Which is fine in and of itself, except, as the authors put it, it's often accompanied by an implicit assumption: "therefore, we no longer have to do Y." And Y, in these cases, was the scientific grunt work involved with showing a given correlation is relevant, general, driven by a mechanism we can define, and so forth.
And the reality is that the grunt work is so hard that a lot of it is never going to get done. It's relatively easy to use a computer to pick out thousands of potentially significant differences between the human and mouse genomes. Testing the actual relevance of any one of those could occupy a grad student for a couple of years and cost tens of thousands of dollars. Because of this dynamic, a lot of the insights generated using big data will remain stuck in the realm of uncertainty indefinitely.
Recognizing this is probably the surest antidote to the problem of big data hubris. And it might help us think more clearly about the sorts of big data work that are most likely to make a lasting scientific impact.
Science, 2014. DOI: 10.1126/science.1248506 (About DOIs).
reader comments
46